Exploring Meta Robyn and Google Lightweight: Open-Source MMM Packages
As the field of marketing analytics evolves, the role of marketing mix modeling (MMM) has become increasingly prominent in helping businesses optimize their marketing strategies. Two open-source packages—Meta’s Robyn and Google’s Lightweight MMM—have gained significant attention among analytics professionals. While both tools have empowered marketers with free, flexible solutions, they are not without limitations. This article explores the advantages, drawbacks, and areas for improvement of these popular MMM packages, especially from the perspective of media agencies and marketers without technical expertise.
Meta Robyn: A Robust Yet Complex Tool
Meta Robyn, developed by Meta (formerly Facebook), has earned praise for its robustness and scalability. Built on R, this package leverages evolutionary computing to model the impact of various marketing channels. It provides advanced features like multi-objective optimization, which allows users to balance ROI with incremental sales.
Advantages of Meta Robyn
- Advanced Modeling Capabilities: Meta Robyn supports complex modeling scenarios, making it suitable for businesses with diverse and intricate marketing data.
- Optimization Features: The multi-objective optimization functionality helps identify the best marketing mix for achieving various goals.
- Transparency and Customization: As an open-source tool, Robyn’s algorithms and processes are transparent, allowing experienced data scientists to customize and adapt it to their specific needs.
Drawbacks of Meta Robyn
- Steep Learning Curve: The reliance on R programming and evolutionary computing can be daunting for marketers without a technical background.
- Performance Concerns: Meta Robyn’s advanced calculations often require significant computational resources, leading to long running times for large datasets.
- Difficulty Aligning with Commercial and Marketing Criteria: Meta Robyn’s algorithm, based on evolutionary computing, struggles to incorporate domain knowledge or pre-determined variable contribution percentages. Users must run the model, check whether variable contributions meet expected criteria, and adjust transformation parameters to re-run as needed.
- Limited Accessibility for Non-Technical Users: Smaller media agencies and independent marketers may find it challenging to implement and interpret results without dedicated data professionals.
Meta Robyn uses multiple-objective optimization driven by evolutionary computing to minimize the difference between share-of-spend and share of variable effect. However, this method has limitations: (1) If the optimized results still do not yield a satisfactory share of effect, how should users address this issue? (2) Non-media variables, such as socio-economic factors, are not tied to spend and cannot be quantified in dollars—how should users handle the share of effect for these non-media variables? The following screenshot illustrates how closely the share-of-spend and share-of-effect align, highlighting the optimization process.
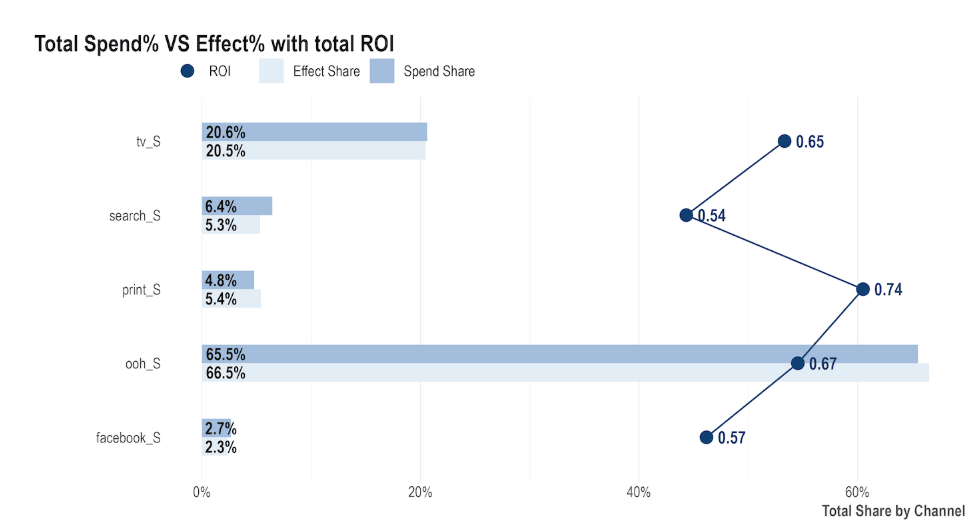
Google Lightweight MMM: Simplicity with Limitations
Google’s Lightweight MMM is another popular option in the open-source MMM space. Developed in Python, this package focuses on providing a simpler, more accessible approach to marketing mix modeling while maintaining the accuracy of its results, based on Bayesian regression.
Advantages of Google Lightweight MMM
- Ease of Use: With its focus on simplicity, Lightweight MMM offers a lower barrier to entry for users familiar with Python.
- Efficiency: Designed for faster calculations, it delivers results more quickly compared to Meta Robyn, making it suitable for agile marketing environments.
- Open-Source Flexibility: As an open-source package, it allows for customization to meet unique business requirements.
Drawbacks of Google Lightweight MMM
- Steep Learning Curve: The reliance on Python programming and Bayesian regression can be daunting for marketers without a technical background.
- Performance Concerns: Advanced calculations, particularly for Markov Chain Monte Carlo simulations, often require significant computational resources, leading to long running times for large datasets.
- Difficulty Aligning with Commercial and Marketing Criteria: The algorithm’s reliance on prior distribution of variable coefficients makes it challenging to align variables and their contribution percentages with expected criteria. Users must iterate by adjusting prior distributions and re-running the model to meet predefined goals.
- Technical Barrier: Although easier to use than Robyn, it still requires Python expertise, leaving non-technical marketers at a disadvantage.
Google Lightweight adjusts the prior distribution, including its shape and parameters, to influence the variable share effect. This can be a challenging setting, even for data analytics professionals. The following screenshot demonstrates how a Python file needs to be modified using a half-normal distribution to ensure the media variable has a positive impact. This method also requires multiple attempts to achieve the final satisfactory results.
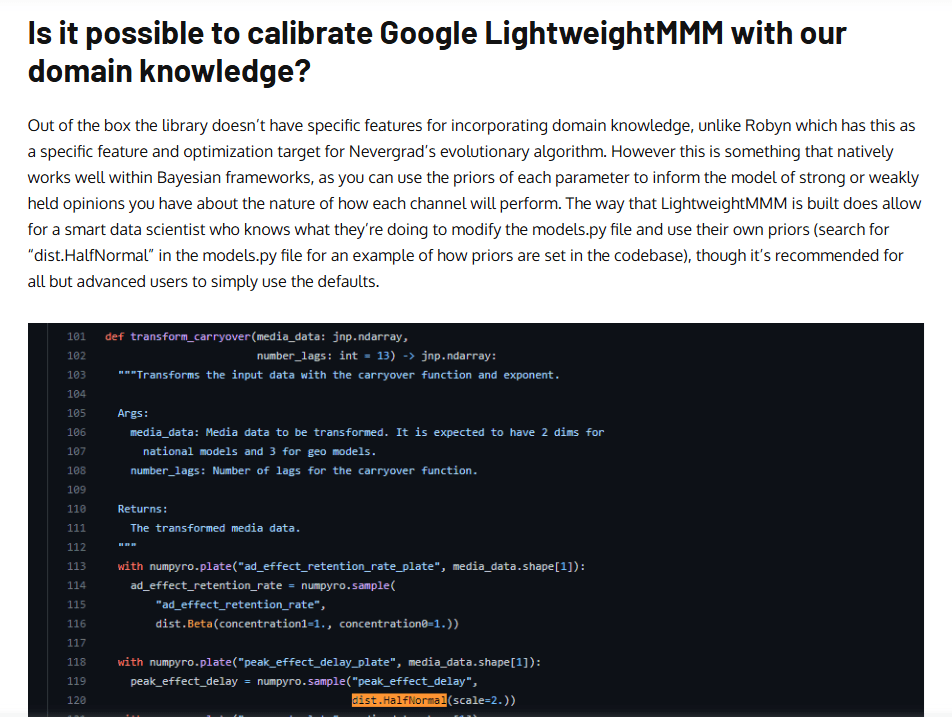
Challenges for Smaller Media Agencies and Non-Technical Marketers
Despite their open-source nature, both Meta Robyn and Google Lightweight MMM face significant barriers when it comes to adoption by smaller media agencies and marketers without technical expertise. These challenges include:
- Technical Expertise Requirements: Both tools require proficiency in programming languages (R for Robyn and Python for Lightweight MMM) and statistical modeling, which are not typically part of a marketer’s skill set.
- Running Time: Meta Robyn’s resource-intensive computations and Google Lightweight MMM’s relatively faster but still technical processes can make adoption challenging for teams with limited computational power or time.
- Customization Challenges: While both packages are customizable, doing so often requires a level of technical skill that smaller agencies and independent marketers lack.
- Alignment with Business Goals: Neither tool is designed with an out-of-the-box capability to accommodate specific commercial and marketing criteria, which can limit their usability for strategic decision-making.
Opportunities for Improvement
To make these tools more accessible and practical for a wider range of users, several enhancements could be considered:
- No-Code Solutions for Non-Technical Users: Developing tools that do not require programming knowledge would significantly expand accessibility.
- User-Friendly Interfaces: GUI-based versions of these tools could lower the barrier to entry for non-technical users.
- Integrated Training Resources: Comprehensive tutorials, case studies, and user guides can help users gain confidence in using these tools.
- Faster Computation Solutions: Migrating to cloud platforms can reduce running times and computational resource requirements, making the tools more efficient for smaller agencies.
- Built-In Business Rules: Incorporating features that allow users to easily apply commercial and marketing criteria would improve alignment with business objectives. For example, enabling users to set variable contribution percentages before modeling and guiding the model evolution to meet predefined criteria.
Conclusion
Meta Robyn and Google Lightweight MMM represent significant advancements in open-source marketing mix modeling. Their capabilities have empowered many marketing analytics professionals to build robust models and gain valuable insights. However, their limitations in terms of running time, technical accessibility, and alignment with commercial and marketing criteria cannot be overlooked.
For smaller media agencies and marketers without technical expertise, the complexity and resource requirements of these tools remain significant hurdles. By addressing these challenges through user-friendly enhancements, cloud-based solutions, and improved training resources, these open-source packages could become even more impactful. Until then, businesses must carefully weigh the benefits and drawbacks to determine if these tools are the right fit for their marketing analytics needs.
We are More Than Data, and we are here to help media agencies fulfill the need for user-friendly, fast-to-run, easy-to-use tools that align with commercial and marketing criteria. Our sophisticated software, Minute MMM, is designed to make media measurement accessible and effective for all.