Building MMM the Traditional Way: Why It’s So Time-Consuming
In our previous article, Why Small and Medium-Sized Media Agencies Haven’t Used MMM, we discussed some barriers preventing agencies from adopting Marketing Mix Modeling (MMM). Another significant reason is the time-consuming nature of building MMM using traditional methods. Let’s explore why this process takes so much time.
Slow Data Collection
Data collection is one of the biggest bottlenecks in traditional MMM processes. For digital channels like search, social media, and online advertising, gathering data is relatively quick and straightforward. However, offline channels—such as TV, Out-of-Home (OOH), and radio—are a completely different story.
Offline data often arrives in inconsistent formats, mingled across Excel and PDF files with ever-changing table structures and headings. For example, one file might label OOH data as “outdoor,” while another uses “out of home.” While the meaning is clear, the lack of standardization creates delays as teams must spend significant time reconciling and cleaning the data.
If the data comes in a PDF format, it often leaves us questioning—how do we even begin to extract the numbers accurately? Take this example from a client case: A media agency received a 90-page PDF from Channel 9 after booking TV ads. Despite requesting an Excel version, none was provided, and attempts to use online PDF-to-Excel converters failed to produce accurate results, leaving the agency frustrated and overwhelmed. That’s where we stepped in. Leveraging our software, we transformed the PDF into a clean, structured Excel file, turning an otherwise daunting task into a seamless process.
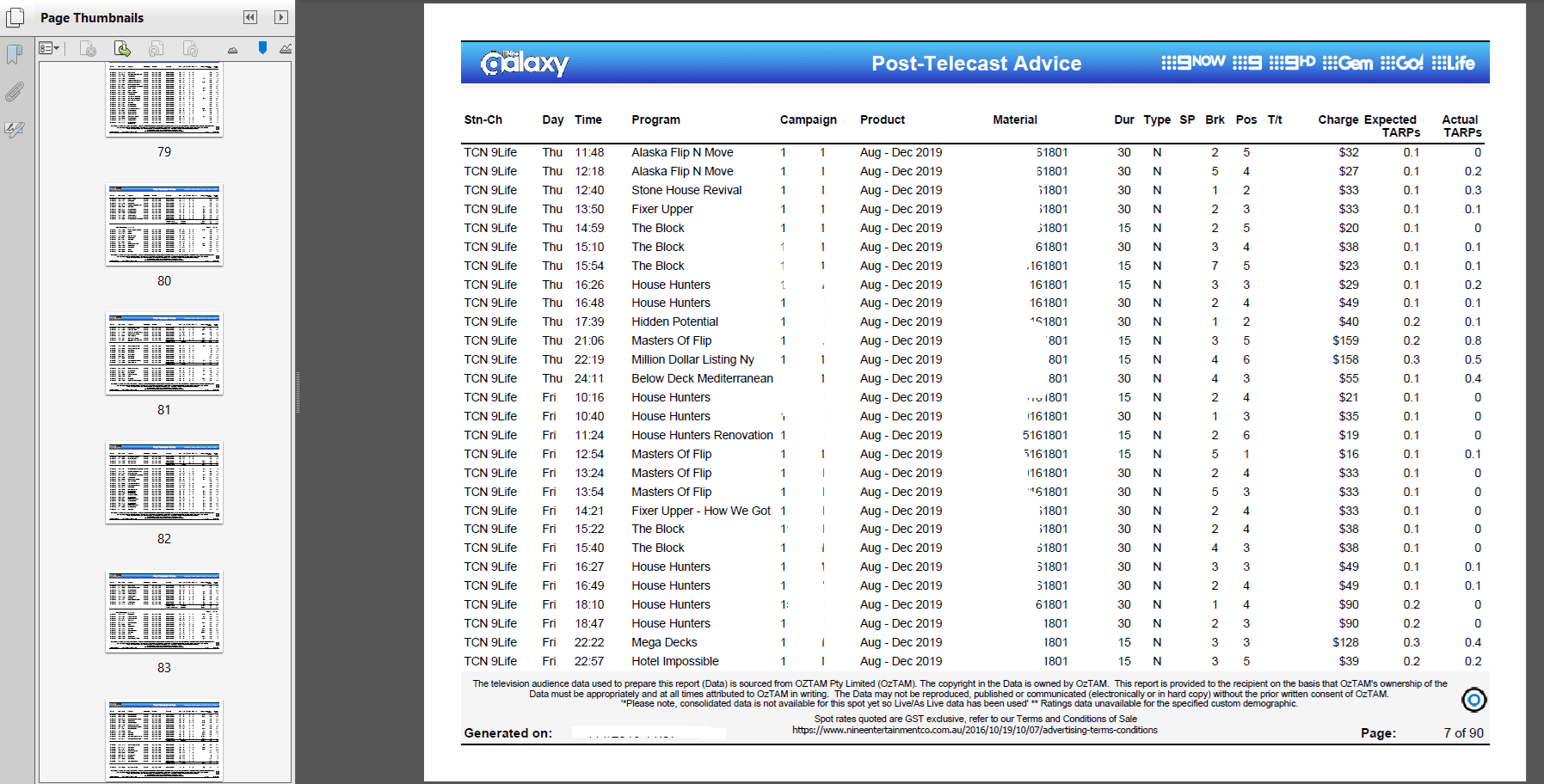
Slow Data Processing
Even after the data is collected, processing it can be another hurdle. Many media agencies rely on Excel for this step, using pivot tables and manual indexing. This manual work is prone to errors and inefficiencies. While some advanced users leverage VBA (Visual Basic for Applications) for automation, most marketers find it too complex and time-consuming to learn and implement effectively.
Excel VBA enables powerful data processing through coding and workflow design. While it can present a learning curve, many non-technical users and media agencies have successfully leveraged it to streamline their data operations with the right guidance and resources. Should additional support be needed, we are always here and happy to assist.
Furthermore, while R and Python are widely used in data analytics and processing, the key consideration is how non-technical users and media agencies can be empowered to effectively leverage these programming languages to achieve their goals. And, if additional support is needed, we are always here and ready to assist.
Tedious Data Quality Checks and Validation
Data validation often becomes an endless loop of back-and-forth updates. Agencies frequently revise datasets multiple times, signing off on one version only to find errors that require new updates.
In one of our experiences with a media agency, we collaborated on tracking 10+ iterations of a dataset over six months. These updates reflected the evolving nature of their operations, including adjustments to media spend, special offers, competitor activity, and even KPI sales figures, which were refined four times. This dynamic environment underscores the complexity of maintaining a stable foundation for effective MMM while adapting to real-world changes.
Complex Model Building Process
Once the data is processed and validated, building the MMM itself introduces additional complexities. The model must satisfy both commercial and marketing criteria, as well as adhere to statistical assumptions and tests.
In this article, we focus solely on commercial and marketing practice criteria. Readers interested in the statistical aspects can refer to the previous article, Building MMM the Traditional Way: Why It’s Way Too Expensive.
For example, in the FMCG industry, agencies might estimate that TV contributes 5% of sales, paid social 3%, paid search 1–2%, and OOH 6%. Meanwhile, discounts and promotions account for 12% and 11%, respectively. These percentage-based criteria require the modeler to validate each media variable one by one, transforming raw media spend data using techniques like diminishing returns and adstocking.
However, the process is highly sensitive: adjusting one variable often disrupts the balance of others, requiring constant recalibration. This iterative cycle can feel like a frustrating game of "whack-a-mole," where solving one issue creates new ones.
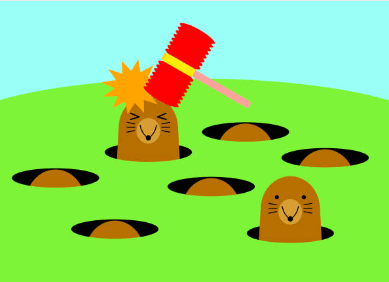
Why This Matters
Traditional MMM methods are simply too slow and inefficient for fast-paced scenarios. For instance, if a media agency needs to create a small-scale pitch model to win a new client, the lengthy MMM process makes it impossible to meet tight deadlines.
As a result, many agencies opt to partner with freelancers for specialized expertise or focus on highlighting their strengths in creative capabilities and the experience of their teams in their pitch decks. While data-driven insights may not always be the focal point, agencies often incorporate carefully selected data visuals or dashboard screenshots to demonstrate their understanding of analytics and provide a comprehensive view of their approach.
Conclusion
The traditional way of building MMM is plagued by inefficiencies at every step—data collection, processing, validation, and modeling. For media agencies to truly embrace MMM, they need faster, more streamlined solutions that align with the time-sensitive nature of their work.
If this resonates with your challenges, why not chat with us at More Than Data? We’re here to help! Our solutions streamline the MMM process, addressing inefficiencies in data collection, processing, validation, and modeling. With our expertise, you can focus on delivering impactful insights without the headaches of traditional methods.
Let’s work together to make your data-driven marketing a reality—quickly and effectively. Contact us today!